“As the sun dipped low in the western sky, casting long shadows against the sleepy town of St. Petersburg, I found myself strolling lazily along the banks of the Mississippi River.”
“A broad expanse of the river was turned to blood; in the middle distance the red hue brightened into gold, through which a solitary log came floating.”
One of these passages is by Mark Twain. The other is written by ChatGPT in the style of Twain. Can you tell the difference? If not, what might that mean?
These were some of the questions that inspired a recent project by Gabi Kirilloff, assistant professor of English, and Claudia Carroll, a postdoctoral research associate with the Transdisciplinary Institute in Applied Data Sciences (TRIADS). This summer, with support from the Humanities Digital Workshop, Kirilloff and Carroll assembled an interdisciplinary team of undergraduate and graduate students to explore literary bias and style in GPT, a type of artificial intelligence model that processes and generates human-like text.
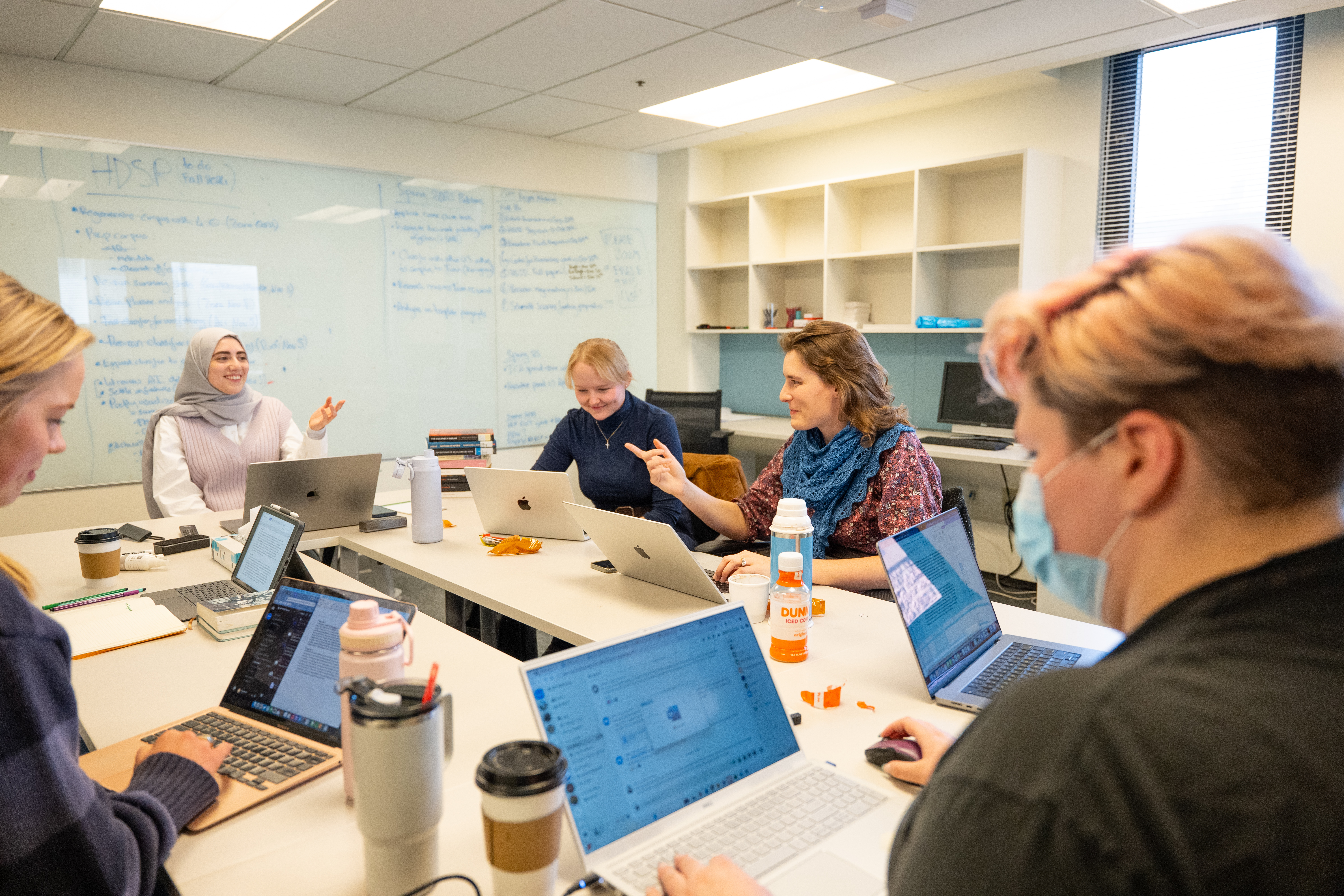
With the rapid rise of large language models such as GPT (which generated the first passage about “sleepy St. Petersburg”), the question has never been more relevant.
Kirilloff completed her PhD training in English at the University of Nebraska-Lincoln, studying under Matthew Jockers, a pioneer in computational text analysis and cultural analytics. With her interest and experience in combining qualitative and quantitative work, Kirilloff saw an opportunity to use data science to consider how gender bias plays out in the literary canon. Based on anecdotal evidence, GPT seemed better at replicating classical male authors than female ones, she said. This initial hypothesis spurred a data-driven study to validate these impressions and see what other tendencies AI might be able to reveal.
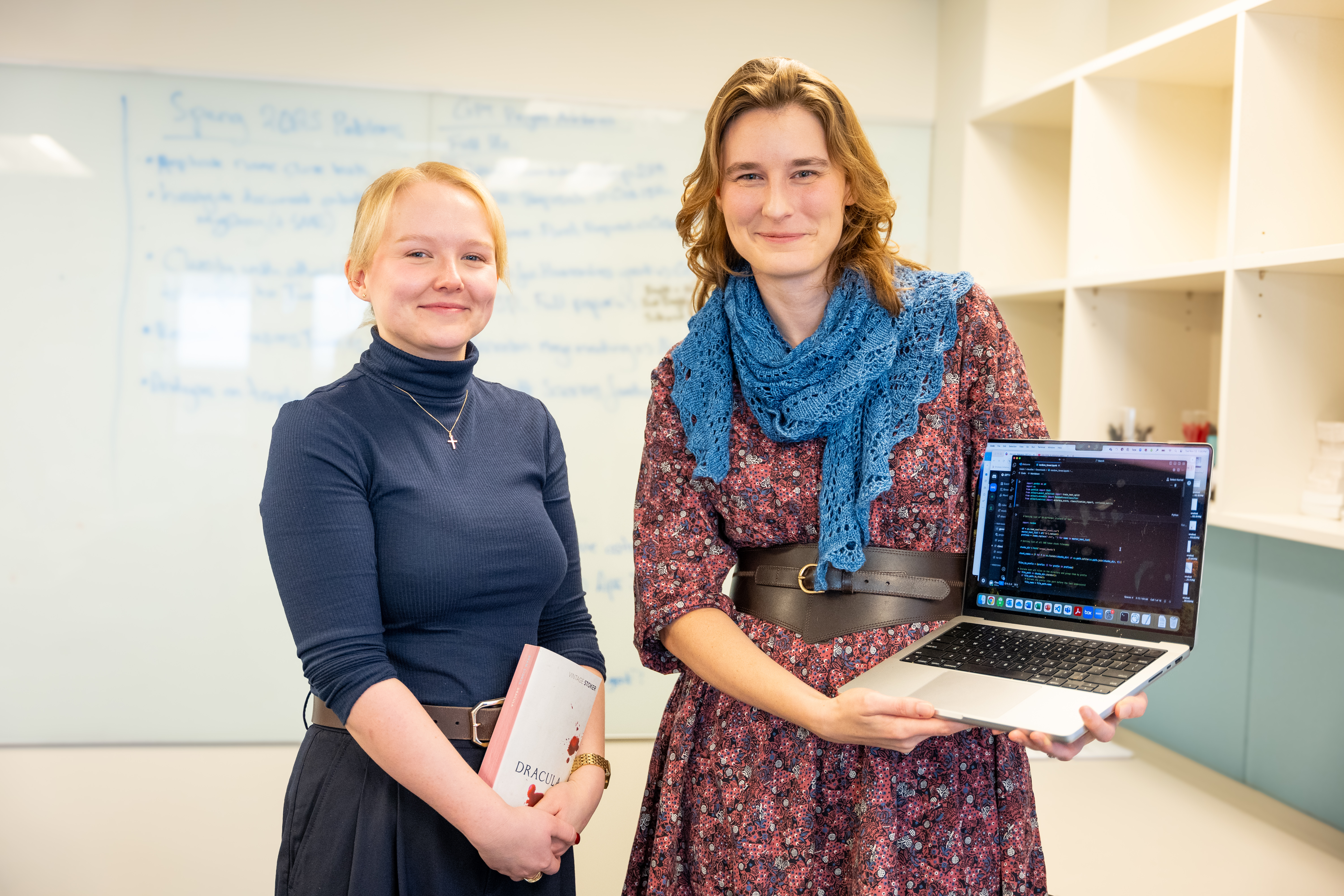
Carroll, a self-taught coder who began using programming for her English PhD research at Notre Dame, suspected analyzing GPT's training data would uncover hidden biases. “The training data we have comes from what people considered ‘important’ literature,” Carroll said. “Who gets to decide what’s important is often determined by privilege and wealth. There are institutional and structural reasons why the data available to train AI models is determined by issues of cultural prestige and power.”
Another motivating factor for the project was Kirilloff's observation of the range of public perceptions about AI. While many data scientists she talked to were enthusiastic about GPT, she often encountered suspicion from colleagues in the humanities. To her, both perspectives were important. “I see many who are critical of AI and have a lot of anxiety about it,” Kirilloff said. “But one of the ways to make that space productive is to do projects like this, where we are given a sense of agency to interrogate it and try to make sense of it as a tool.”
Joseph Loewenstein, professor of English and director of the Humanities Digital Workshop, said the project plays a critical role in shaping our understanding of AI. “ChatGPT is maturing rapidly and while philosophers and data scientists have inspected the way it ‘thinks,’ Kirilloff and Carroll are probing its stylistic aptitudes and clumsinesses,” he said. “I’m thrilled that they’re turning the tools of computational stylometry on ChatGPT itself, taking its measure rather than letting it take the measure of others.”
Navigating uncharted territory
While Kirilloff and Carroll began discussing the project in early 2024, work officially began over the summer when the Humanities Digital Workshop supported the project with computer science and humanities students serving as fellows.
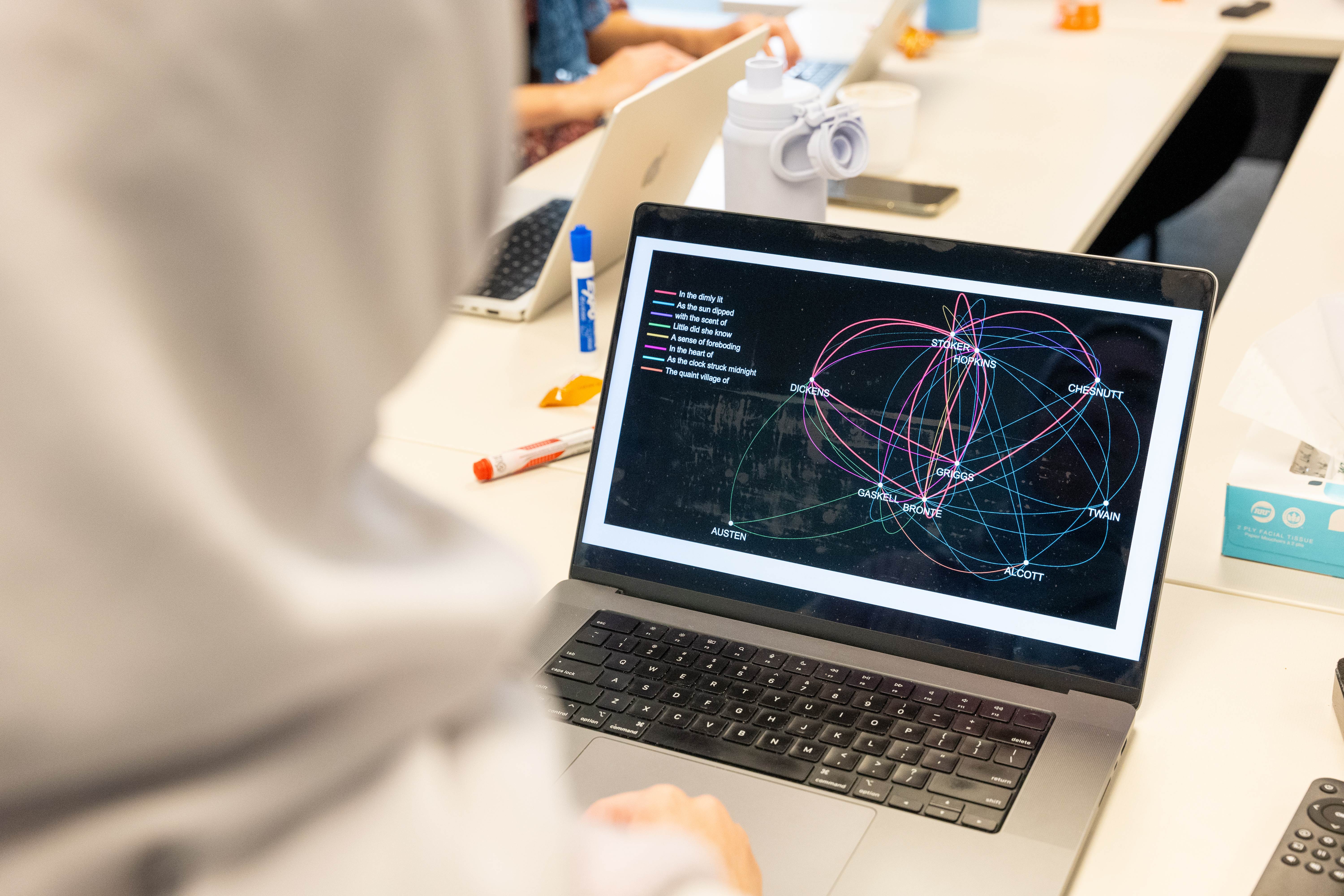
The team began by picking 10 popular authors from the 19th-century canon, choosing a careful mix of male and female, Black and white, American and international writers. They settled on Jane Austen, Pauline Hopkins, Louisa May Alcott, Elizabeth Gaskell, Charlotte Brontë, Charles Dickens, Charles Chesnutt, Mark Twain, Sutton Griggs, and Bram Stoker.
Then, it was time to involve GPT. The team asked the AI model to produce original works in the style of each author.
At first, the team struggled to craft prompts that would elicit meaningful responses from GPT. Prompt writing is an emerging field, Kirilloff said, and the best practices are still being developed. “It was overwhelming at first," she said, "but also exciting to be in uncharted territory."
The next step was to develop a classifier, a data science term for a model or an algorithm that assigns labels to data. Their classifier used 207 features such as sentence length, word choice, and word frequency to determine if a text was authentic or generated by GPT.
The classifier analyzed more than 40,000 pieces of writing, and the best model achieved 99% accuracy in predicting which texts were authentic and which were synthetic. “I was surprised that the accuracy of our classifier was so high,” Kirilloff said. “We’re not totally sure why this is.”
The analysis also identified a major outlier: Mark Twain. The GPT simulations of Twain were far more likely to be misclassified as authentic than those produced in the style of any other author. GPT, they found, was surprisingly good at imitating Twain.
The reasons for this are not entirely clear, but Kirilloff and Carroll have a few theories. One possibility is that unlike other well-known authors on their list — Austen, Brontë, and Dickens, for instance — Twain doesn’t occupy the same space in popular culture. The other authors have sparked plenty of fan fiction, Goodreads reviews, and contemporary recreations of their works. (Think “Pride Prejudice and Zombies,” the 2009 novel by Seth Grahame-Smith, or the countless versions of “A Christmas Carol”.) Most online language associated with Twain, on the other hand, is actually by Twain. The researchers termed this “noise theory” and will seek to test it, along with several other theories, in the next phase of their project.
“There’s been the assumption that with large language models, the more training data about an author the better,” Kirilloff said. “But this research points to the fact that it’s not necessarily about more and more data; it's about the quality of the data. In some cases, the more training data that is likely available for an author, the worse the results.”
Keeping pace with the rapid growth of GPT
Kirilloff and Carroll attribute some of the project’s success to their lab research model, a method more common in the natural sciences than the humanities. Their team includes students from computer science, data science, media studies, and comparative literature. The student fellows have played a crucial role in shaping the project's intellectual trajectory, they said.
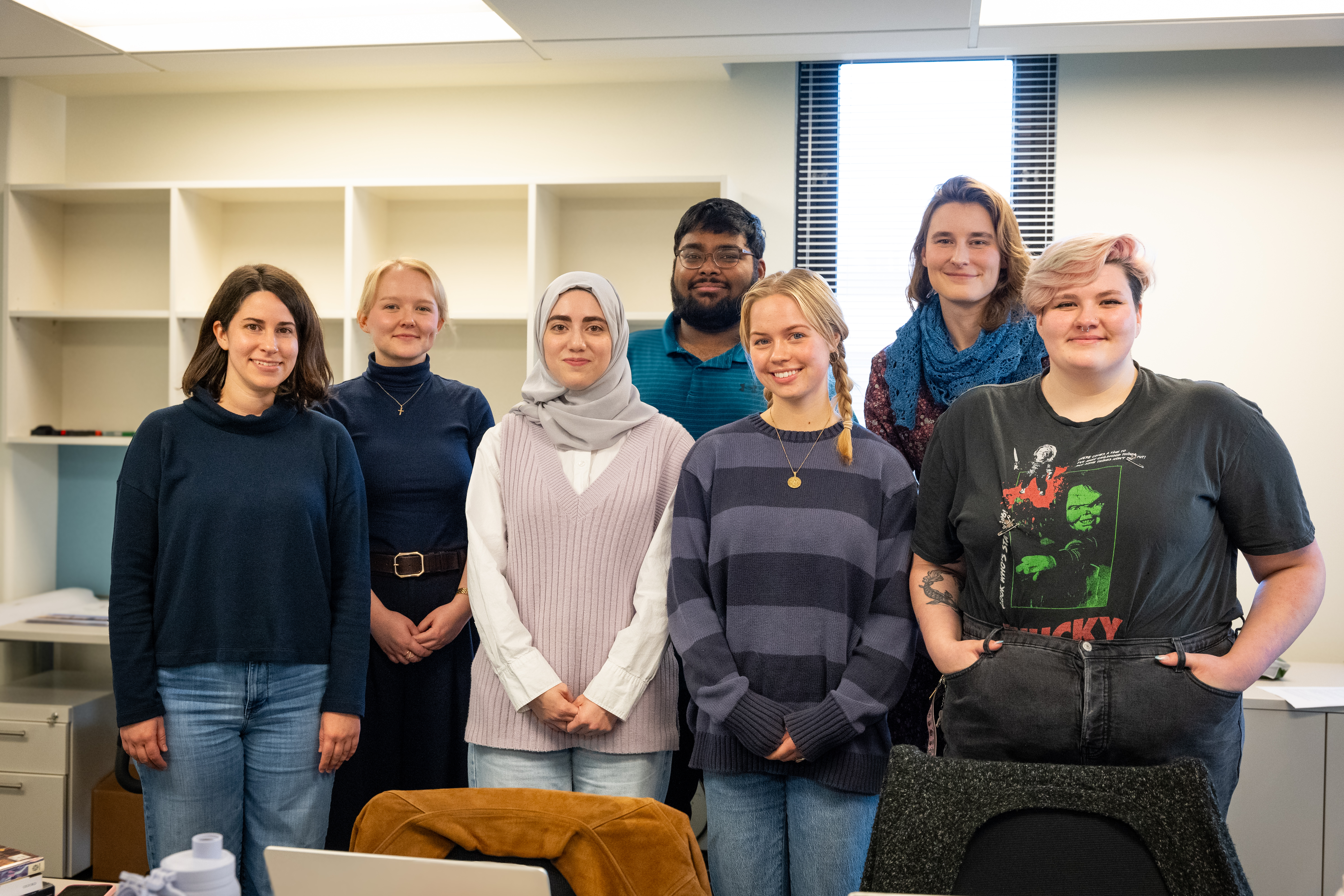
“It’s not us doing all the thinking and then delegating to them,” Carroll said. “The students are genuinely helping direct the research, raising questions, and thinking of methods we aren’t as familiar with in our discipline.”
Razi Khan, a senior majoring in computer science and economics, has been a part of the lab for two semesters. Along with his majors, he’s pursuing a data science in the humanities (DASH) certificate, designed to provide cross-disciplinary exposure between the humanities and computer science.
After graduation, Khan hopes to oversee a technical team, a job that would call on the skills he’s learned in this project. “Gaining a deeper understanding of how large language models work has been valuable, and the interdisciplinary interactions I've experienced have helped me communicate more clearly, avoiding jargon when talking to people outside the computer science field,” Khan said.
Moving forward, the team aims to test their theory about Twain and diversify their pool of source authors. Because white authors traditionally dominate the literary canon, they anticipate their model will excel at imitating them, even if more authors of color are added. But Carroll is already thinking of other ways to analyze diversity.
“We want to think in more depth and detail about how GPT is manifesting race, not just in terms of what kinds of representations GPT produces, but how it produces them in terms of style markers like grammar and syntax,” she said. The team also plans to incorporate more contemporary works, though they anticipate some challenges related to copyright restrictions.
The group’s first paper proposal has been accepted and they are currently working on a draft. Still, the rapid pace of change in AI means the team will always have more to explore and more challenges to tackle.
“We are trying to keep up with the pace that things are changing,” Kirilloff said, “but it’s valuable to take a snapshot in time to understand historically how these models operated — even if things are different a year from now.”