The Department of Statistics and Data Science is preparing to leverage the insights of scholars across WashU to make artificial intelligence more trustworthy.
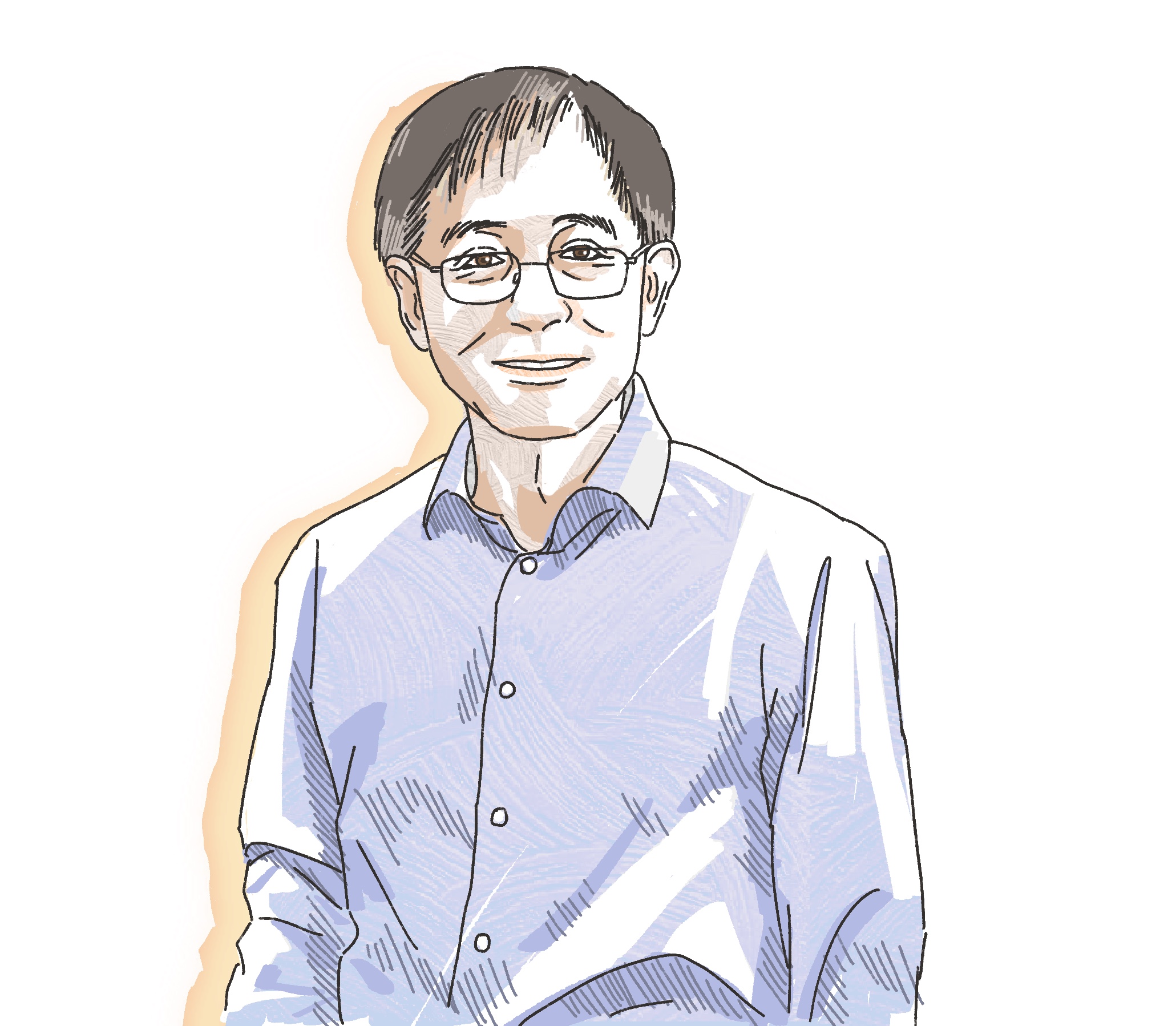
We’ve all seen the power of artificial intelligence, and not just when we’re interacting with a customer service chatbot or looking for help with a writing project. Tech companies are already mapping out a future where AI becomes an inescapable part of our daily lives, and governments are embracing the technology with both urgency and caution. In just a few short years, AI has transformed fields as diverse as economics, medicine, and climatology.
In short, AI is here to stay. As the inaugural chair of the Department of Statistics and Data Science, it’s my job to work with WashU faculty and students to make AI more ethical, accurate, fair, and, yes, intelligent.
At this pivotal point in history, we should take a step back and consider the true nature of AI. At its core, the technology is built on statistical ideas and algorithms. Every choice made by AI — selecting words for an essay, generating pixels for an image, directing turns for a self-driving car — starts with data. Algorithms churn through huge data sets to make informed decisions. But it’s statisticians who are the experts, investigating and understanding the underlying process and its inherent uncertainties.
To appreciate how statistics and AI intersect, consider one of the key challenges for data scientists: subgroup analysis. This approach — a focus of my own research — examines how different groups of people respond to a particular intervention. Take the world of medicine: In clinical trials, subgroup analysis can help determine if a treatment is effective for certain groups of patients. As the data dimensions increase (think about all the intersections of age, gender, race, medical history, and more), so does the number of potential subgroups to consider. Computer-powered AI could theoretically find the metaphorical needle in a haystack — a group of patients who could benefit from a specific treatment. But before we celebrate, a question must be asked: Did we find a real needle or merely an artificial one?
Misuse of subgroup analysis can have serious consequences. Consider the 2002 case of a biotech firm that announced that an FDA-approved immunosuppressant could reduce mortality from idiopathic pulmonary fibrosis (IPF). That would have been a breakthrough, but a federal jury later found that the firm’s CEO had cherry-picked trial data to find a significant result. AI advancements make it easier than ever to find seemingly impressive results, amplifying the risk of selective reporting.
That’s where statisticians come in. We’re tasked with understanding how the data are collected and how subgroups are selected so that we can honestly evaluate the findings. This takes more than just computing power.
It's my job to work with WashU faculty and students to make AI more ethical, accurate, fair, and, yes, intelligent.
Without proper input from statisticians, AI could be seriously biased. To be clear, AI itself is not biased by design, but it does reflect the underlying data. If the data aren’t fair and representative, and if analysts lack the statistical sophistication to interpret their findings, the results could be extremely off-base. Here’s another example: In 2018, the American Civil Liberties Union tested Amazon's AI-based facial recognition technology by having it compare photos of federal lawmakers against a database of publicly available mug shots. The AI falsely matched 28 members of Congress with people who had been arrested, and a disproportionate percentage of those bad matches were people of color.
As statisticians and data scientists, we work to mitigate these biases to help AI reach the right conclusions. Through thoughtful experimental designs, informative model building, and careful control of spurious correlations, we can help ensure that AI works for everyone.
Indeed, this is an exciting time for statisticians. Academic departments like ours can’t rival Google or Apple for building new AI products, but we do have an important role. We recognize that a smart, safe future for AI will require cross-disciplinary collaboration between industry, government, and academia.
The Department of Statistics and Data Science is preparing to build closer ties with researchers in public health, environmental sciences, business, economics, bioscience, and social science to ensure we have the AI tools to address the complex questions in those fields.
While the future is indeed brighter with AI, the future of AI depends on us.
Xuming He is the Kotzubei-Beckmann Distinguished Professor and chair of the Department of Statistics and Data Science. He is president of the International Statistical Institute and a renowned leader in the fields of robust statistics, quantile regression, Bayesian inference, and post-selection inference.